History Of AI in Healthcare From Beginning to 2025
Published: 04 Apr 2025
AI in healthcare has come a long way. Today, it helps doctors to diagnose diseases, predict health risks and even assist in surgeries. But AI in medicine is not new, it has been evolving for decades.
The journey began in the 1950s when scientists first explored whether machines could think like humans. Over the years, AI systems have grown from simple rule-based programs to complex models that analyze medical images, process patient data and even suggest treatments.
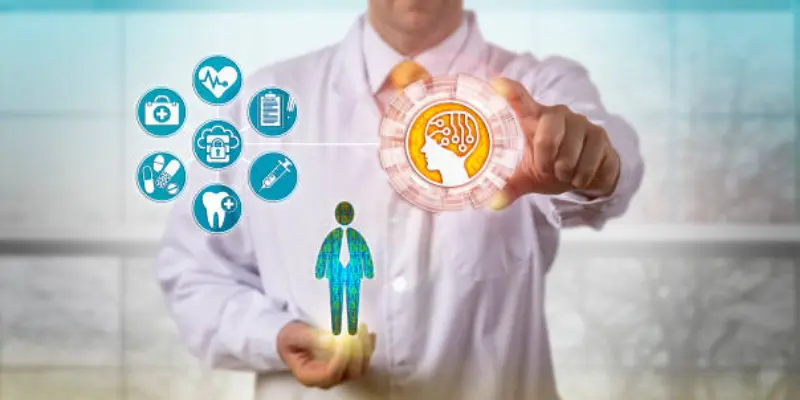
This post will take you through the history of AI in healthcare, from its early days to its present impact. You will learn about key milestones, groundbreaking AI systems and how technology shaped modern medicine.
The Early Years: AI’s First Steps in Healthcare
AI in healthcare didn’t start overnight. Scientists began exploring the idea of intelligent machines decades ago. In the early years, AI was simple and limited, but it laid the foundation for the advanced medical AI we use today.
1950s-1960s: The Birth of AI Concepts
In the 1950s, computer scientist Alan Turing introduced the idea that machines could think like humans. This sparked interest in artificial intelligence. Around the same time, early AI researchers started developing programs that could solve problems using logic.
By the 1960s, the first AI models were designed for medical use. These systems aimed to help doctors in analyzing patient symptoms and suggest possible conditions. However, computers were slow and AI was not advanced enough to make accurate predictions.
1970s: Expert Systems and Rule-Based AI
The 1970s brought a major shift in AI with the rise of expert systems—programs that used “if-then” logic to make decisions. One of the most famous early healthcare AI systems was MYCIN, developed at Stanford University.
How MYCIN Worked:
- MYCIN helped doctors to diagnose bacterial infections like meningitis.
- It asked questions about a patient’s symptoms and suggested possible treatments.
- The system worked well but was never widely used because doctors didn’t fully trust AI yet.
💡 Why Early AI Faced Challenges:
- Computers were slow and expensive.
- AI needed large amounts of data, which was hard to collect.
- Many doctors preferred traditional methods over AI recommendations.
Even with these challenges, these early systems direct the way to modern AI in medicine. The next step was improving AI’s ability to learn from data, which led to even bigger advancements in the 1980s and beyond.
1980s-1990s: Growth of AI in Medical Decision-Making
AI in healthcare took big steps forward in the 1980s and 1990s. Computers became faster and AI systems started using more data to improve medical decision-making. While challenges remained, this period helped AI move closer to real-world medical applications.
Advancements in AI Models
During the 1980s, researchers built more advanced decision-support systems. These programs analyzed patient data and helped doctors to make better choices. One well-known system was INTERNIST-I, developed to help diagnose complex diseases.
🔍 How INTERNIST-I Worked:
- It stored medical knowledge and compared patient symptoms to possible diseases.
- Doctors entered patient data and the system suggested likely diagnoses.
- It worked well for certain cases but struggled with rare conditions.
Another breakthrough came with neural networks, a type of AI that mimics the human brain. Researchers began using these networks to recognize patterns in medical data, which improved disease prediction and diagnosis.
Challenges AI Faced in This Era
Even with these advancements, AI in healthcare still had obstacles:
Cons |
---|
❌ Limited computing power made AI slow and expensive. |
Despite these challenges, the groundwork was laid for future AI breakthroughs. The next big leap came in the 2000s, when AI became more data-driven and started making a real impact in hospitals.
2000s-Present: AI’s Rapid Growth in Healthcare
AI in healthcare advanced quickly in the 2000s as computers became faster and digital health records became common. AI moved from simple rule-based systems to data-driven models that could analyze large amounts of medical information. In the 2010s and 2020s, AI-powered tools started assisting doctors, improving patient care and even helping in drug discovery.
2000s: Machine Learning and Data-Driven AI
The early 2000s saw a shift from rule-based AI to machine learning, where computers learned from medical data instead of following fixed rules.
🔹 Key Advancements:
- Electronic Health Records (EHRs): Hospitals started using digital records, giving AI access to more patient data.
- AI for Disease Prediction: AI systems analyzed medical histories to predict risks for conditions like diabetes and heart disease.
- Early AI Imaging Tools: AI-assisted radiology tools began identifying abnormalities in X-rays and MRIs.
2010s: Deep Learning and Breakthroughs
The 2010s brought major improvements in AI, thanks to deep learning—an advanced form of machine learning that helps AI recognize patterns in complex data.
✅ IBM Watson Health (2011): IBM Watson was one of the first AI systems designed to help doctors diagnose and treat diseases. Although it had limitations but it showed AI’s potential in healthcare.
✅ AI in Radiology & Pathology: AI-powered tools could detect cancer in medical images with high accuracy.
✅ AI for Personalized Medicine: AI helped in tailoring the treatments based on a patient’s genes and health history.
✅ AI-Assisted Robotic Surgery: Robots powered by AI, like the da Vinci Surgical System, started assisting surgeons in complex procedures.
2020s: AI’s Role in Global Healthcare
In the 2020s, AI became even more advanced, playing a key role in global healthcare challenges.
COVID-19 and AI’s Impact:
Positive Impacts |
---|
|
🚀 Other Advancements:
- Virtual Health Assistants: AI chatbots like Babylon Health and Ada Health started helping patients with basic medical advice.
- AI-Powered Drug Discovery Startups: Companies like Insilico Medicine, BenevolentAI and Atomwise used AI to speed up drug development.
What’s Next?
AI is now a key part of modern healthcare, but the journey is far from over. The next big step is AI-powered hospitals, fully automated diagnostics and better AI regulations to ensure patient safety.
The Biggest AI Breakthroughs in Healthcare
AI has brought game-changing advancements to the healthcare industry. From detecting diseases early to speeding up drug discovery, AI has made medical care more efficient and precise. Here are some of the most groundbreaking AI breakthroughs that have transformed healthcare.
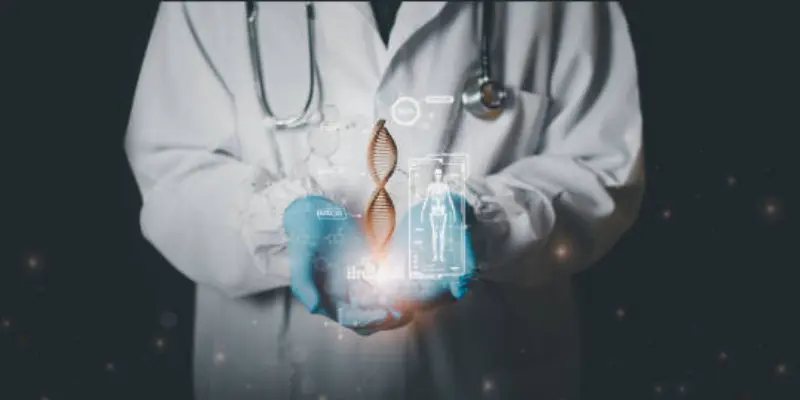
AI in Medical Imaging and Diagnosis
📌 How AI is improving medical scans:
- AI systems can analyze MRIs, X-rays and CT scans to detect diseases like cancer, strokes and fractures.
- Tools like Google’s DeepMind AI can identify eye diseases with accuracy matching top doctors.
- AI-assisted diagnosis speeds up early disease detection and leads to faster treatments and better survival rates.
💡 Example: AI in mammograms possibly can detects breast cancer 5 years earlier than traditional screenings.
AI for Drug Discovery and Development
Developing new drugs takes years but AI is speeding up the process.
🚀 Key AI advancements in drug discovery:
- AI can scan millions of chemical compounds to find new drug candidates in weeks instead of years.
- Companies like Atomwise and BenevolentAI use AI to develop treatments for cancer, Alzheimer’s and rare diseases.
- AI models like DeepMind’s AlphaFold, solved the protein folding problem which is necessary for designing new medicines.
💡 Example: During the COVID-19 pandemic, AI helped researchers identify potential drugs and vaccines much faster.
AI-Powered Robotics in Surgery
How AI is transforming surgeries:
- AI-powered robotic systems like da Vinci Surgical System assist doctors in performing minimally invasive procedures.
- AI improves surgical precision, leading to faster recovery and fewer complications.
- Some AI robots can even operate without human intervention in basic procedures.
💡 Example: AI-assisted robotic surgery has reduced post-surgical complications by 30% in some hospitals.
AI in Personalized Medicine and Patient Care
AI helps create customized treatments based on a person’s unique medical history.
📊 What AI can do:
- AI analyzes a patient’s genetic data, lifestyle and medical history to suggest the best treatment.
- AI-driven virtual health assistants provide 24/7 medical support for answering patient queries and also reminding them to take medications on time.
- AI tools have the ability to predict disease risks, allowing early intervention and better health outcomes.
💡 Example: AI is now used to personalize cancer treatments, ensuring patients get the most effective therapy for their condition.
The Future of AI in Healthcare
AI has already transformed healthcare but its future promises even bigger advancements. As technology improves, AI will play an even greater role in diagnosing diseases, personalizing treatments, predicting rare diseases and improving patient care.
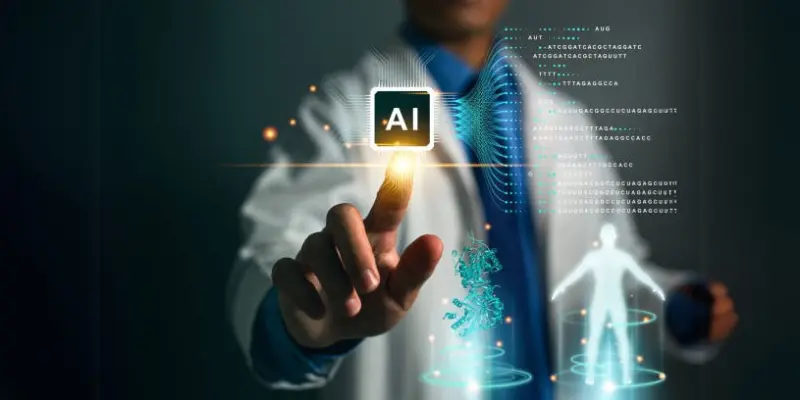
AI-Powered Hospitals and Smart Healthcare Systems
Hospitals of the future will rely on AI-driven automation to improve efficiency and reduce errors.
🏥 What to Expect:
- AI-Enhanced Diagnostics: AI will detect diseases faster and more accurately than ever. For example, AI models are expected to identify cancers at their earliest stages.
- AI-Managed Patient Records: AI will streamline medical records and reduce paperwork which will help the doctors to access patient histories instantly.
- Robotic AI Assistants: AI-powered robots will assist in surgeries and even handle routine patient care tasks.
Personalized Medicine and AI-Driven Treatments
Every patient is different. AI will make treatments more personalized by analyzing individual health data.
How AI Will Help:
- Precision Medicine: AI will suggest the best treatment plans based on a person’s genes and medical history.
- AI-Powered Drug Discovery: AI will speed up new drug development and will help in finding treatments for rare and complex diseases.
- Predictive Healthcare: AI will analyze health trends to predict diseases before they happen, allowing for early intervention.
Ethical Challenges and AI Regulations
With AI becoming more powerful, it also raises concerns about privacy, bias and decision-making. The future of AI in healthcare depends on how well these issues are addressed.
⚖️ Key Challenges:
- Data Privacy: Protecting patient information will be a top priority.
- Bias in AI: AI systems need diverse and unbiased data to ensure fair medical decisions.
- Regulations: Governments and healthcare organizations must create rules to ensure AI is safe and effective.
Key Takeaways: How AI Has Transformed Healthcare
AI has a long journey in healthcare, from early expert systems to advanced deep learning models. It has changed the way doctors diagnose and predict diseases, develop treatments and provide patient care. Let’s look at the major takeaways from AI’s journey in healthcare.
AI’s Milestones in Healthcare
📌 1960s-1980s: The Birth of Medical AI
- Early expert systems like MYCIN and Dendral helped with disease diagnosis.
- AI was limited due to slow computers and lack of digital medical data.
📌 1990s-2000s: The Rise of Machine Learning
- AI moved from rule-based systems to data-driven models.
- Electronic Health Records (EHRs) helped AI analyze patient data.
- AI-assisted radiology tools started detecting diseases in medical images.
📌 2010s-Present: AI’s Rapid Growth
- Deep learning revolutionized medical imaging and diagnostics.
- AI-powered chatbots, virtual assistants and robotic surgeries became common.
- AI played a major role in COVID-19 response and helped with disease tracking and vaccine research.
The Biggest AI Breakthroughs in Healthcare
AI in Medical Imaging– AI now detects cancers, fractures and brain diseases in scans with high accuracy.
AI for Drug Discovery– Companies like Atomwise and BenevolentAI use AI to speed up medicine development.
AI-Powered Robotics– AI-driven robots assist surgeons in complex surgeries, improving precision and patient outcomes.
Predictive AI– AI helps predict diseases early, allowing doctors to take preventive action.
✅ What’s Next for AI in Healthcare?
- More personalized treatments based on genetic data.
- AI-powered hospitals that automate administrative and medical tasks.
- Smarter AI assistants to help doctors and reduce their workload.
Conclusion
So guys, in this article, we covered the history of AI in healthcare in detail. From expert systems in the 1960s to AI-powered robots and predictive analytics today, the impact of AI on medicine is undeniable. The big question now is: What’s next? AI is still developing and its future in healthcare is full of possibilities.
💡 My recommendation: Whether you’re a doctor, researcher or just curious about AI, keep learning about AI’s latest breakthroughs—they’re shaping the future of medicine!
📢 Want to stay ahead? Subscribe to our newsletter for more insights on AI in healthcare!
Related Queries about Healthcare AI History
Here are frequently asked questions about AI Healthcare History;
AI’s journey in healthcare began in the 1960s with early expert systems like Dendral and MYCIN, which were designed to help with medical diagnosis. However, AI’s impact grew significantly in the 2000s and beyond with advancements in machine learning and deep learning.
One of the first AI systems in healthcare was MYCIN, developed in the 1970s at Stanford University. It was designed to help doctors diagnose bacterial infections and recommend treatments.
Early AI in healthcare used rule-based expert systems, which relied on predefined “if-then” logic. By the 2000s, AI began using machine learning to analyze data which allowed computers to learn and improve without explicit programming. Today, AI uses deep learning for tasks like medical imaging and disease prediction.
In the 2010s, AI became widely used in radiology, pathology and robotic surgery. AI systems like IBM Watson Health were developed to assist doctors with diagnosis, while deep learning models improved cancer detection in medical imaging.
AI played a very important role in tracking the virus, predicting outbreaks and accelerating vaccine development. AI-driven chatbots also helped to provide medical advice to patients, reducing the burden on healthcare professionals.
Some well-known AI healthcare systems include:
- IBM Watson Health – Used for analyzing medical data and assisting in diagnosis.
- DeepMind’s AlphaFold – Revolutionized protein structure prediction for drug discovery.
- AI-powered radiology tools – Help detect diseases like lung cancer and stroke from medical images.
AI adoption was slow due to limited computing power, lack of digital medical data and skepticism among doctors. The rise of electronic health records and improvements in AI models allowed wider adoption in the 2000s.
AI speeds up drug discovery by analyzing vast amounts of biomedical data and identifying potential drug candidates faster. Drug AI Companies like Insilico Medicine and BenevolentAI use AI to find treatments for diseases that previously took years to research.
Some key challenges include:
- Data privacy concerns – Protecting patient information from misuse.
- AI bias – Ensuring AI models are trained on diverse datasets for fair medical decisions.
- Regulatory issues – Governments need to establish clear guidelines for AI in medicine.
AI will continue to evolve, leading to fully AI-powered hospitals and more accurate disease predictions. In the future, AI might even play a role in preventing diseases before they occur!